Financial institutions, including both banks and insurers, have been facing rising levels of financial crime for a long time, particularly cyber crime. This is especially true during the pandemic. Alongside this we have a tightening regulatory framework around anti money laundering, know-your-customer requirements, and counter terrorism funding regulations, as well as more general regulatory changes including the new General Insurance Code of Practice and the new AFCA complaints and Internal Dispute Resolution requirements in Australia.
There is an increasing workload required of financial institutions to find, investigate, and report fraudulent activity, as well as an increasing variety of methods used by fraudsters, and greater risk of loss to the organisation. Added to this, the sheer volumes of data being produced in an increasingly measured and online world has been both a blessing and a curse depending on how it’s collated and used.
This has seen a growing number of solutions aimed at leveraging datasets to detect fraud, often with particular specialities – transactions, loan applications, insurance claims, and so on. This can lead to a very siloed approach in some institutions, particularly banks where the nature of their operations requires a greater variety of detection systems. It can also come about because teams have been created at different times for different reasons – e.g. an anti-fraud team was built to help customers and reduce losses, but an AML/KYC team might have come about due to regulatory change.
There are a couple of problems with this situation. One is fairly straightforward – expense. Running multiple teams for different reasons, especially with little mutual aid or communication, is very expensive. Each team may have duplicates of other teams’ cases, each team has its own management structure, its own paperwork, and so on.
The other, more important situation is co-ordination. Financial crimes are complex and evolving, and may touch on numerous aspects of your operations. In the above situation of siloed AML/KYC teams and fraud investigation teams, organised crime syndicates that may be picked up by AML/KYC have a risk of being involved in fraud rings. If your teams are not sharing information, you may miss a red flag like a contact number from a KYC case showing up on a potential fraud. Similarly, you may miss details from a transaction fraud case that appear on a loan application, or the account a claim is to be paid into may go to a bank account that’s been flagged for AML.
The curse of big data is the combination of the above two issues – if you fail to unify and analyse all your various sources of data, you will be snowed under, and with little useful insight to show for it.
As such, there has been an increasing focus on unified financial crime systems within financial institutions. An approach often discussed is the ‘hub and spoke’ model, where numerous data sources are collated in one central location for one team to look at, and potentially one analytics solution to run over the data. However, this may not work for some institutions for the reasons mentioned above – data sources are quite varied, and a transaction monitoring engine may not be suitable for application or insurance fraud, or vice versa. Additionally, the project costs required for replacing multiple existing solutions may be prohibitive.
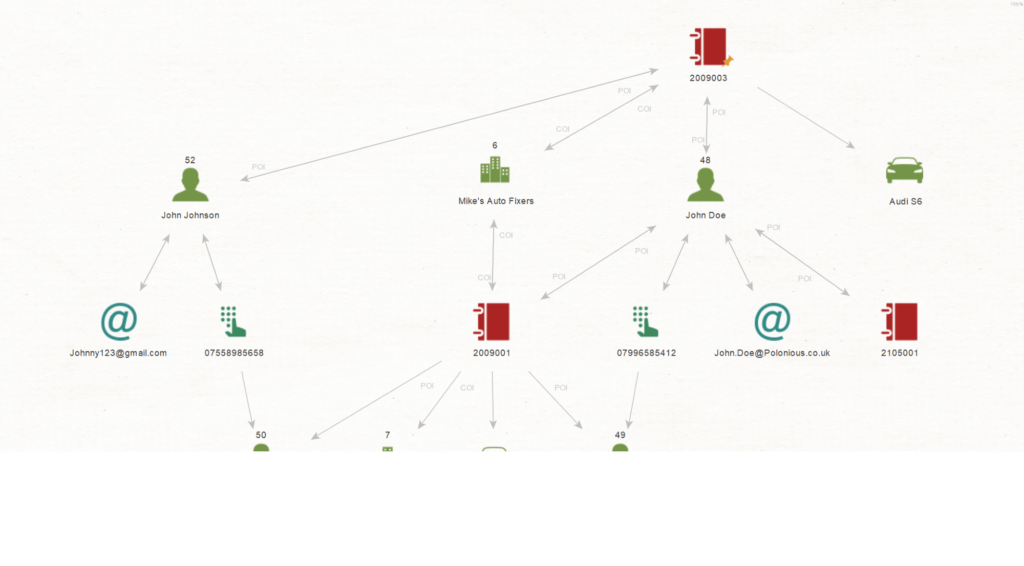
So, what do all of these potential fraud cases have in common? Well, they need to be investigated. Whether it’s to triage and potentially report to regulators in the case of AML, to recover money from another financial institution in a card fraud case, or to prepare a brief for recovery via the courts, all of these cases require some level of investigation. For reasons we’ve outlined before, analytics engines alone will not provide a holistic fraud solution, not to mention the issues with operating across different data sets.
As such, the natural place to collate all of the insights various detection and analytics systems can provide, and without the project costs of replacing several existing systems, is to add an integrated investigation management system as the hub to which all of your intelligence and analytics spokes connect.
Modern integrations via tools such as APIs allow seamless transfer of flagged cases between detection or analytics systems and a case manager, meaning you can run a smaller team that works only in the case manager itself. Two way integration means that updates can be fed back to detection and analytics systems, improving their accuracy, as well as passing new information between systems via the hub.
APIs also make the integration process easier, making it possible for systems such as Polonious to integrate with multiple leading analytics and detection solutions with minimal fuss.
Bringing every red flag into the one system then allows you to lay other intelligence tools over the top, such as graphical link analysis, to provide even greater insight across your whole dataset.
Lastly, all of these red flags and potential investigations are brought into a system which is specifically designed for robust investigations which comply with all your regulatory requirements.
Financial and cyber criminals are becoming increasingly sophisticated. Running a bureaucratic and fractured anti-fraud and anti money laundering program simply will not keep up.
Let's Get Started
Interested in learning more about how Polonious can help?
Get a free consultation or demo with one of our experts
Nick Fisher
After being a client of Polonious while working in the Student Discipline team at Curtin University in Perth, Nicholas joined Polonious as a systems configurer in 2017. He since completed many successful rollouts as well as building Polonious' marketing team. Nicholas became CEO of Polonious when the founders retired in 2023.
Nicholas holds a Bachelor of Commerce, and a Bachelor of Arts with Honours in Philosophy from the University of Western Australia.